**Embrace Imperfections:** Even The Best Simulations Won't Be Perfect. Treat Them As Estimations, Not Predictions.
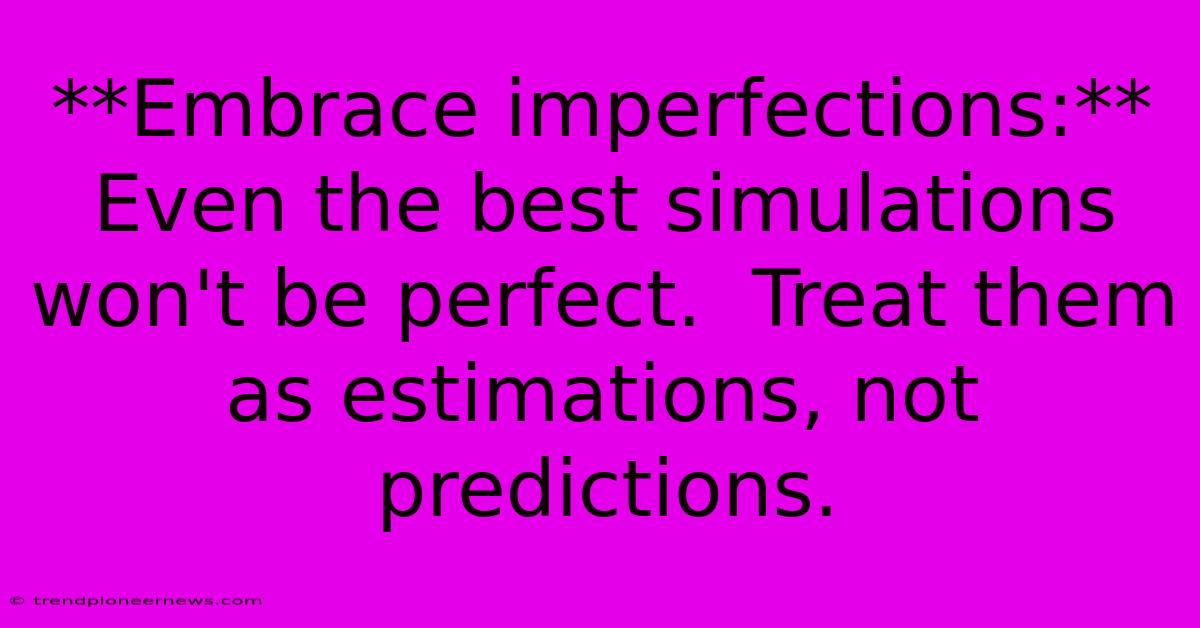
Discover more detailed and exciting information on our website. Click the link below to start your adventure: Visit Best Website **Embrace Imperfections:** Even The Best Simulations Won't Be Perfect. Treat Them As Estimations, Not Predictions.. Don't miss out!
Table of Contents
Embrace Imperfections: Even the Best Simulations Won't Be Perfect
Hey everyone, let's talk about something that's been bugging me – and probably you too – for years: the nagging feeling that our simulations, no matter how sophisticated, aren't perfect. And you know what? They're not supposed to be. I used to beat myself up about it, chasing that elusive perfect model. Let me tell you, that's a rabbit hole, my friend. A deep, dark rabbit hole.
My Simulation Snafu: The Case of the Missing Penguins
I remember one project, a wildlife population model I was super proud of. I spent months on it, tweaking parameters, adding variables, even incorporating that crazy new algorithm I read about. I thought it was going to be the best thing since sliced bread. I was convinced it would accurately predict penguin populations over the next decade. My simulation showed a steady increase, a beautiful, upward trending line. I even presented it at a conference! Felt pretty darn smart, let me tell ya.
Then, reality hit. Hard. Field data showed a sharp decline. Turns out, I'd missed a crucial factor: a change in the ocean currents affecting their primary food source. My complex model, with all its bells and whistles, had failed to capture a simple, fundamental aspect. It was humbling, to say the least. I felt like a total idiot.
The Big Lesson: Estimations, Not Predictions
That experience taught me a huge lesson: treat your simulations as estimations, not predictions. Simulations are powerful tools for understanding complex systems, but they're not crystal balls. They're built on assumptions, simplifications, and, let's face it, incomplete data. There's always gonna be something you miss. Always.
Thinking about it now, I should have done more background research, considering factors beyond population dynamics. A thorough literature review combined with additional data collection techniques might have shed light on the effect of the ocean currents. I had the tools, but I didn’t use them correctly.
Actionable Insights: How to Deal With Imperfect Simulations
So, how do we deal with this inherent imperfection? Here’s what I do now:
-
Embrace Uncertainty: Acknowledge the limitations of your model upfront. Don't try to hide the uncertainties; highlight them. It shows you understand the limitations of your work, which actually boosts credibility.
-
Sensitivity Analysis: Run multiple simulations, changing key parameters to see how sensitive your results are. This helps to quantify uncertainty and identify the most influential factors. This also helps with debugging!
-
Validation and Verification: Don’t just rely on theoretical models. Continuously validate your results against real-world data. This is crucial in improving the accuracy of your model over time.
-
Iterative Improvement: Simulations are an iterative process. Expect to refine your model over time as you gather more data and improve your understanding of the system.
-
Collaboration: Talk to experts in the field. Get feedback on your model and assumptions. A fresh perspective can identify blind spots you may have missed.
It's also super important to remember that the value of a simulation often lies in the process itself—the insights gained during the model’s development, not just the final output. In my case, even though the penguin prediction was off, the process identified crucial environmental factors that could impact populations. That's still valuable data.
Remember, perfection is the enemy of good enough. Focus on making your simulations as accurate as you can, given the available data and resources, and be transparent about the uncertainties involved. Then, get ready to learn and iterate! Trust me, it's a much less stressful way to work. And who knows, maybe you'll even discover some seriously cool things along the way!
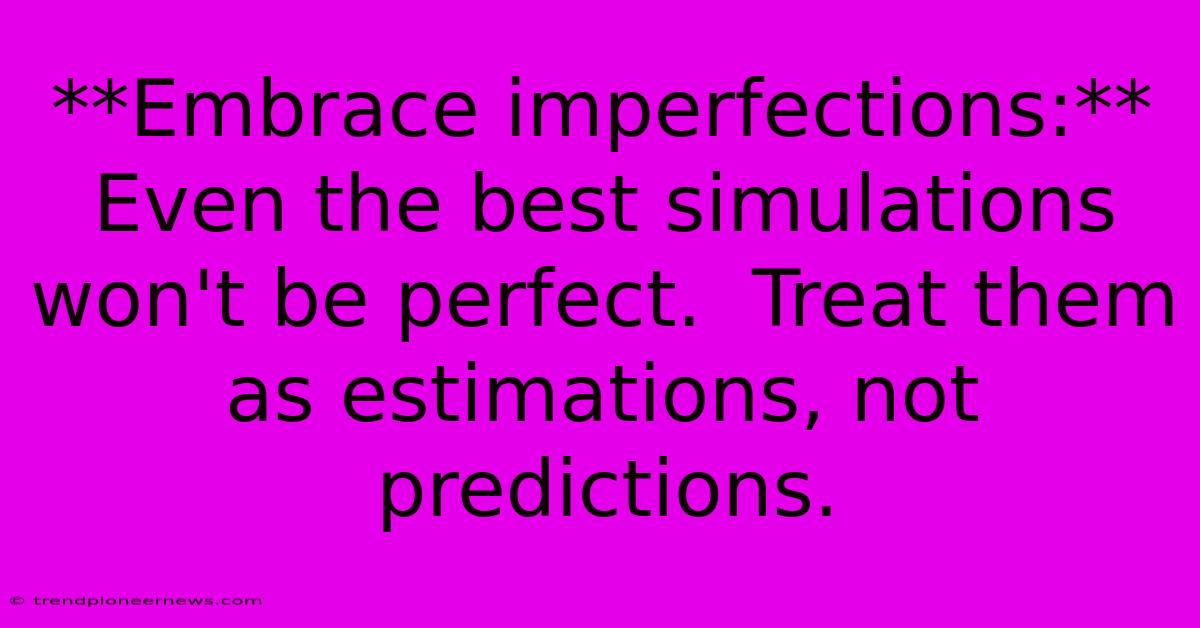
Thank you for visiting our website wich cover about **Embrace Imperfections:** Even The Best Simulations Won't Be Perfect. Treat Them As Estimations, Not Predictions.. We hope the information provided has been useful to you. Feel free to contact us if you have any questions or need further assistance. See you next time and dont miss to bookmark.
Featured Posts
-
Aviation Experts Key To Airline Success
Nov 27, 2024
-
Trump Tariffs Hidden Costs
Nov 27, 2024
-
Molly Verdict Department Acknowledges Mistake
Nov 27, 2024
-
Proper No Twelve Whiskey New Source
Nov 27, 2024
-
Congress Forgives 3 B In Student Debt
Nov 27, 2024